Abstract
Aims/hypothesis
Young children who develop multiple autoantibodies (mAbs) are at very high risk for type 1 diabetes. We assessed whether a population with mAbs detected by screening is also at very high risk, and how risk varies according to age, type of autoantibodies and metabolic status.
Methods
Type 1 Diabetes TrialNet Pathway to Prevention participants with mAbs (n = 1815; age, 12.35 ± 9.39 years; range, 1–49 years) were analysed. Type 1 diabetes risk was assessed according to age, autoantibody type/number (insulin autoantibodies [IAA], glutamic acid decarboxylase autoantibodies [GADA], insulinoma-associated antigen-2 autoantibodies [IA-2A] or zinc transporter 8 autoantibodies [ZnT8A]) and Index60 (composite measure of fasting C-peptide, 60 min glucose and 60 min C-peptide). Cox regression and cumulative incidence curves were utilised in this cohort study.
Results
Age was inversely related to type 1 diabetes risk in those with mAbs (HR 0.97 [95% CI 0.96, 0.99]). Among participants with 2 autoantibodies, those with GADA had less risk (HR 0.35 [95% CI 0.22, 0.57]) and those with IA-2A had higher risk (HR 2.82 [95% CI 1.76, 4.51]) of type 1 diabetes. Those with IAA and GADA had only a 17% 5 year risk of type 1 diabetes. The risk was significantly lower for those with Index60 <1.0 (HR 0.23 [95% CI 0.19, 0.30]) vs those with Index60 values ≥1.0. Among the 12% (225/1815) ≥12.0 years of age with GADA positivity, IA-2A negativity and Index60 <1.0, the 5 year risk of type 1 diabetes was 8%.
Conclusions/interpretation
Type 1 diabetes risk varies substantially according to age, autoantibody type and metabolic status in individuals screened for mAbs. An appreciable proportion of older children and adults with mAbs appear to have a low risk of progressing to type 1 diabetes at 5 years. With this knowledge, clinical trials of type 1 diabetes prevention can better target those most likely to progress.
Similar content being viewed by others
Avoid common mistakes on your manuscript.
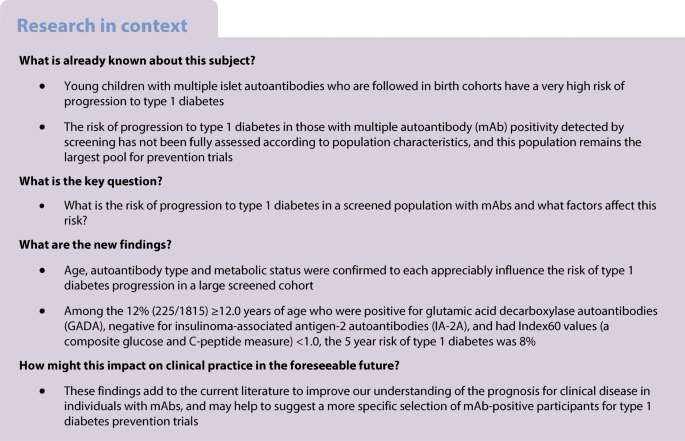
Introduction
Findings from birth cohorts of young children at high familial or genetic risk for type 1 diabetes have shown that those who develop multiple autoantibodies (mAbs) are at very high risk for type 1 diabetes [1,2,3,4,5]. In fact, it has been proposed that the presence of mAbs is already indicative of the eventual progression to clinical type 1 diabetes [5, 6]. However, cohorts of individuals with mAbs that are identified by screening might not have the same risk for several reasons. For example, a vast majority of their positive autoantibodies are prevalent rather than incident. Also, the risk of type 1 diabetes is inversely associated with age [2, 7] and cohorts screened for autoantibodies are appreciably older than birth cohorts. Moreover, screened cohorts might differ in autoantibody types and metabolic status; either or both could influence type 1 diabetes risk.
There is a need to determine whether the likelihood of progression to type 1 diabetes is also high in screened cohorts with mAbs. High-risk estimates for type 1 diabetes could have a considerable emotional impact and influence the willingness of individuals to enter prevention trials. Importantly, accurate risk estimation is critical for developing correct entry criteria for type 1 diabetes prevention trials.
Several thousands of children and adults who are relatives of individuals with type 1 diabetes have been identified as having mAbs through screening in the Type 1 Diabetes TrialNet Pathway to Prevention (PTP) study. These individuals are followed for diagnostic surveillance of type 1 diabetes with repeat oral glucose tolerance testing (OGTT). This information has provided the opportunity to confirm previous findings and assess the extent that type 1 diabetes risk varies specifically in children and adults with mAbs.
Methods
Participants
Data from the Type 1 Diabetes TrialNet PTP study were utilised [8]. Participants in the PTP study are relatives of individuals with type 1 diabetes. For inclusion in our analysis, individuals required the presence of multiple (≥2) autoantibodies on at least one laboratory draw, found at a screening visit. Participants either had mAbs at enrolment or became positive later, though the vast majority were the former. Islet autoantibody testing (starting in 2001) was initially assessed by radioimmunoassay for glutamic acid decarboxylase autoantibodies (GADA), insulin autoantibodies (IAA) and insulinoma-associated antigen-2 autoantibodies (IA-2A) [9]. Zinc transporter 8 autoantibodies (ZnT8A) [10] were assessed (by radioimmunoassay) if any of the three autoantibodies initially tested were positive at screening. ZnT8A testing was initiated in January 2012, with limited testing from 2004–2008, and participants were included only if they had complete testing for all four autoantibodies of interest. Participants were followed in the TrialNet PTP study for the development of type 1 diabetes with 2 h OGTTs performed every 6 months. Participants with normoglycaemia or dysglycaemia (fasting glucose 5.5–6.9 mmol/l; 30, 60 or 90 min glucose ≥11.1 mmol/l; or 2 h glucose 7.8–11.0 mmol/l) were able to enrol. Those with OGTTs at baseline indicative of diabetes (fasting glucose ≥7.0 mmol/l or 2 h glucose ≥11.1 mmol/l) or those missing OGTT time-point data at baseline (at the start of OGTT monitoring) were excluded. A minimum of one follow-up monitoring visit was required. BMI values obtained within 9 months of the baseline OGTT were used if missing at baseline. A diagnosis of type 1 diabetes in the TrialNet PTP study was made if an OGTT was in the range for diabetes and this was confirmed on repeat testing. If a repeat OGTT was no longer in the diabetes range, participants continue to be followed. Additionally, a clinical diagnosis was made (i.e. symptomatic hyperglycaemia). Informed consent and assent, where applicable, was obtained for all study participants and all participating clinical sites have been approved by an institutional review board.
Procedures
Participants were evaluated for the following risk predictors of progression to type 1 diabetes: age, autoantibody type, positivity for 2 vs >2 autoantibodies, normoglycaemia vs dysglycaemia, and Index60 <1.0 or ≥1.0 (Index60 = [0.3695 × (log10[fasting C-peptide])] + [0.0165 × 60 min glucose] – [0.3644× 60 min C-peptide]). Based on both glucose and C-peptide measurements, Index60 has been used as a metabolic marker of progression to type 1 diabetes [11, 12]. An Index60 threshold value of ≥1.0 was employed to indicate metabolic impairment [12].
Data analysis
Factors and measures of interest were summarised overall as well as within mAb subgroups (e.g. positive for 2 vs 3–4 autoantibodies). Two-sample t tests (or Wilcoxon rank sum tests for comparisons of small subgroups) and χ2 tests were used to compare continuous and categorical measures between subgroups. Time of progression to type 1 diabetes and cumulative incidence distributions were compared between subgroups using univariate and multivariate Cox regression analysis. Adjustments were made for autoantibody number. Also, interaction terms were utilised to investigate the influence of age, BMI, sex and relationship to proband. Kaplan–Meier and cumulative incidence models were used to estimate risk of type 1 diabetes at specific time points of interest. TrialNet PTP participant mAb status was determined based on IAA, GADA, IA-2A and ZnT8A. SAS software (version 9.4; SAS Institute, Cary, NC, USA) was utilised for computation in the analysis.
Results
The study population of TrialNet PTP participants included 10,020 autoantibody positive individuals, with 4145 confirmed single-autoantibody positive and 5875 mAb-positive participants (electronic supplementary material [ESM] Fig. 1). Individuals were excluded if they did not have complete OGTT data, autoantibody testing data, at least one follow-up visit, or age and BMI data. Individuals were also excluded if they had an OGTT in the diabetes range at baseline. Brief characteristics of those excluded are presented in ESM Table 1. A total of 1815 mAb-positive individuals were included in the analysis, divided into those with 2 (n = 804) and 3–4 (i.e. >2; n = 1011) autoantibodies (GADA, IAA, IA-2A, ZnT8A). Ninety per cent were first-degree relatives of individuals with type 1 diabetes. Baseline characteristics of those included are in Table 1. Individuals included had necessary data for analysis (sex was missing in n = 9). The mean (±SD) follow-up time of the cohort was 2.40 ± 2.35 years. Across the overall cohort, the age and BMI were 12.35 ± 9.39 years and 19.66 ± 5.39 kg/m2, respectively, with 46.3% of participants being female. Those with only 2 autoantibodies were, on average, significantly older (p = 0.0310) than those with >2 autoantibodies, but with similar BMI values (p = 0.4936). There was a similar proportion of female participants among those with 2 autoantibodies and >2 autoantibodies (p = 0.2701).
Age
There was an inverse association between type 1 diabetes and age (continuous variable) in those with mAbs (HR 0.97 [95% CI 0.96, 0.99]; p = 0.0004). This pattern is evident in Fig. 1, which shows a significantly lower cumulative incidence among those ≥12.0 years of age than those <12.0 years of age, with both 2 and >2 autoantibodies, as has been demonstrated in birth cohorts and other screened populations. Specifically, participants who were <12.0 years of age at mAb determination (n = 1145) had an estimated 5 year type 1 diabetes rate of 35% (95% CI 31%, 40%) vs those who were ≥12.0 years of age (n = 670), who had an estimated 5 year type 1 diabetes rate of 22% (95% CI 17%, 28%) (HR 0.62 [95% CI 0.48, 0.80]; p = 0.0002). Among those >18 years of age (n = 253), the 5 year type 1 diabetes rate was low at 15% (95% CI 9%, 24%). These arbitrary categories were used to roughly describe pre-/peri-pubertal from post-pubertal age groups.
Number and type of autoantibodies
The cumulative incidence of diabetes at 5 years of follow-up in those with 2 vs >2 autoantibodies at baseline was 29% and 31%, respectively. We assessed the risk of type 1 diabetes according to the types of autoantibodies that were present (ESM Table 2). In Cox regression models adjusting for the number of autoantibodies (2, 3 or 4), the presence of GADA conferred a lower risk of type 1 diabetes relative to the presence of other autoantibodies (HR 0.60 [95% CI 0.43, 0.85]; p = 0.0038). In contrast, IA-2A positivity conferred a higher risk relative to the presence of other autoantibodies (HR 1.97 [95% CI 1.47, 2.64]; p < 0.0001). IAA and ZnT8A were associated with an increased risk of type 1 diabetes in the univariate setting, but not after adjustment for the number of positive autoantibodies.
Figure 2 focuses on GADA and IA-2A, the lowest risk and highest risk autoantibodies, respectively. It shows the cumulative incidence curves for type 1 diabetes among those with 2 autoantibodies who had either GADA or IA-2A as one of two autoantibodies (those positive for both GADA and IA-2A were excluded). The risk for type 1 diabetes was significantly lower in those positive for GADA as one of the two autoantibodies (in the absence of IA-2A) (HR 0.35 [95% CI 0.22, 0.57]; p < 0.0001). Conversely, those positive for IA-2A (in the absence of GADA) were at significantly increased risk of type 1 diabetes (HR 2.82 [1.76, 4.51]; p < 0.0001). The 5 year risk estimates were 21% vs 60% for GADA-positive (without IA-2A) vs IA-2A-positive (without GADA) participants with 2 autoantibodies (Fig. 2).
Descriptive age differences in autoantibody pairs among those with 2 autoantibodies demonstrate what has previously been shown in birth cohorts: those with IAA (IAA/GADA, IAA/ZnT8A and IAA/IA-2A) were younger (ESM Fig. 2a). The combination of IAA/GADA was most common among those with 2 autoantibodies (41%) and provided the lowest risk of progression at 5 years compared with other autoantibody pairs (p = 0.0003, logrank test). GADA/ZnT8A-positive individuals were the largest group of those who were >12.0 years old (40%) (data not shown).
The three autoantibody pairs with the lowest risk of progression to type 1 diabetes at 5 years were IAA/GADA (17% [95% CI 12%, 25%]), GADA/ZnT8A (26% [95% CI 17%, 38%]) and IAA/ZnT8A (33% [95% CI 14%, 63%]). Those with the highest risk of progression at 5 years were GADA/IA-2A (40% [95% CI 29%, 54%]) and ZnT8A/IA-2A (54% [95% CI 34%, 77%]). The sample size of individuals with IAA/IA-2A positivity was low at 5 years; the 3 year cumulative incidence was 30% (ESM Fig. 2). For the lowest risk group (IAA/GADA; see ESM Fig. 2b) at baseline, a longitudinal analysis demonstrated only 26% (84/327) of individuals developed IA-2A or ZnT8A during follow up. The 5 year risk of progression for those that remained IAA/GADA-positive was 15% compared with 20% for IAA/GADA-positive participants that developed IA-2A or ZnT8A positivity later. Those in the IAA/GADA group who developed IA-2A or ZnT8A were younger (mean ± SD: 7.6 ± 5.3 years of age) at the age of mAb determination than those who remained IAA/GADA positive only during follow up (12.5 ± 10.1 years of age; p < 0.0001).
Metabolic status
The cumulative incidence for type 1 diabetes was lower in those with Index60 values <1.0 vs values ≥1.0 in the overall cohort (HR 0.23 [95% CI 0.19, 0.30]; p < 0.0001). These differences in risk based on Index60 were seen both among individuals with 2 autoantibodies (HR 0.17 [95% CI 0.12, 0.24]; p < 0.0001), and in those with >2 autoantibodies (HR 0.29 [95% CI 0.22, 0.40]; p < 0.0001). For Index60 values <1.0 vs ≥1.0, the 5 year estimated type 1 diabetes risk in those with 2 autoantibodies was 20% vs 68%, respectively, and in those with >2 autoantibodies, it was 22% vs 53%, respectively. Similar patterns of influence were also observed between individuals classified as being normoglycaemic vs dysglycaemic with 2 autoantibodies (HR 0.21 [95% CI 0.15, 0.31]; p < 0.0001) and >2 autoantibodies (HR 0.32 [95% CI 0.24, 0.43]; p < 0.0001). For normoglycaemia vs dysglycaemia, the 5 year estimated type 1 diabetes risk in those with 2 autoantibodies was 20% vs 65%, respectively, and in those with >2 autoantibodies, it was 24% vs 49%, respectively.
The association of type 1 diabetes with age is modified by metabolic status (Fig. 3). Among those with Index60 values <1.0, the cumulative incidence was much lower in those ≥12.0 years of age than those <12.0 years of age (p = 0.0009), whereas, among those with Index60 values ≥1.0, there was no longer a significant difference between the two age groups (p = 0.2555). The interaction of the influence of age group by Index60 was also evident using the multivariable Cox regression model, where there was a significant interaction between age and Index60 (p = 0.0019). Other significant interactions included age and BMI (p = 0.0270), age and first-degree relative status (p = 0.0193), age and sex (p = 0.0445), and sex and Index60 (p = 0.0026) (data not shown).
(a) Cumulative incidence with 95% CI (shaded area) of type 1 diabetes in participants with mAbs with Index60 ≥1.0 (red) or Index60 <1.0 (blue) (p < 0.0001, logrank test). (b, c) Cumulative incidence with 95% CI (shaded area) of type 1 diabetes in participants aged <12 years (red) and ≥12 years (blue) with Index60 values <1.0 (p = 0.0009, logrank test) (b) or ≥1.0 (p = 0.2555, logrank test) (c)
To demonstrate the aggregate impact of age, type of autoantibodies and Index60 on type 1 diabetes risk among those with mAbs, we compared a ‘lowest’ risk group (≥12.0 years with GADA present, IA-2A absent and Index60 values <1.0) with a ‘highest’ risk group (<12.0 years old with GADA present or absent [absent would markedly limit numbers], IA-2A present and Index60 values ≥1.0). There was a large difference in the cumulative incidence of type 1 diabetes between the groups (Fig. 4). The 5 year risk estimate was 61% (number at risk: 239/1815; 13% of the cohort) in the highest risk group, whereas it was only 8% in the lowest risk group. That latter group comprised 12% (225/1815) of the study population.
Cumulative incidence with 95% CI (shaded area) of type 1 diabetes in the ‘highest’ risk group (<12 years, IA-2A present, GADA present or absent and Index60 values ≥1.0; red) compared with the ‘lowest’ risk group (≥12 years, GADA present without IA-2A, Index60 values <1.0; blue) (p < 0.0001, logrank test)
Discussion
Type 1 diabetes is increasingly recognised as being a heterogeneous disorder. This is evident with regard to age of onset [13], genetic diversity [14,15,16,17], differing autoantibody associations [18,19,20] and metabolic differences [21, 22]. Our findings of marked variation in risk among those with mAbs in the TrialNet PTP population is consistent with the heterogeneity of type 1 diabetes. For each of the characteristics studied (age, autoantibody number, autoantibody type and Index60), there were appreciable proportions of individuals who appeared unlikely to progress to type 1 diabetes. Moreover, when these characteristics were combined, a sizeable proportion of individuals were at lower risk compared with those without this combination of characteristics. Even among those with >2 autoantibodies, the ultimate progression to type 1 diabetes was still questionable in a considerable number at 5 years of follow up.
The findings presented here suggest that the risk associated with mAbs differs between cohorts defined by incident autoantibodies (such as birth cohorts) and cohorts defined through screening at-risk relatives of individuals with type 1 diabetes. The latter cohorts are predominantly comprised of those with prevalent mAbs, such as the TrialNet PTP. Incident autoantibodies could be indicative of more aggressive disease. It is possible that a good proportion of prevalent autoantibodies are long-standing and, thus, representative of less progressive disease, especially if first identified in later childhood or adulthood. Our study confirms several findings from birth cohorts and smaller screened populations and adds to the current literature regarding individuals at risk for type 1 diabetes with mAbs, in addition to identifying a subgroup of individuals with relatively low risk of progression.
The inverse association of type 1 diabetes with age has been shown in several cohorts [2, 4, 5, 13, 20]. Data from TrialNet [23] and birth cohorts, such as The Environmental Determinants of Diabetes in the Young (TEDDY) [15], suggest that the association of type 1 diabetes with age is complex and dependent on the timing of the appearance of autoantibodies. Lower risk has been associated with GADA and higher risk has been associated with IA-2A and ZnT8A in autoantibody positive populations; however, most studies have assessed single autoantibodies and mAbs combined [5, 24]. The lower risk conferred by the presence of GADA in a mAb group was also seen in TEDDY, where slower progression to diabetes was demonstrated in children with stable GADA positivity but lacking IA-2A, regardless of age at seroconversion [25]. It should be emphasised that, although GADA was shown to represent a group with lower diabetes risk in our study, it was in the context of a mAb-positive population. Thus, our findings do not negate the evidence that GADA is a risk factor for type 1 diabetes in the general population, and the 5 year risk of diabetes remains higher for individuals with GADA plus another autoantibody compared with those with single autoantibodies [26]. The degree of risk for type 1 diabetes with IA-2A as one of two positive autoantibodies is similar to those with >2 autoantibodies, confirming the high-risk nature of IA-2A seen in birth cohorts and European screened cohort studies [5, 20, 24, 26, 27].
Perhaps the most surprising finding in our study includes the breakdown of risk by autoantibody pairs. Among those with 2 autoantibodies, the 5 year type 1 diabetes risk was only 17% among those with IAA and GADA. Birth cohort participants typically develop IAA or GADA first, based on age, and later, with the development of mAbs, have a high lifetime risk of type 1 diabetes [28]. Our study confirms the Belgian Diabetes Registry findings of Gorus et al [20] in that participants with IAA/GADA had a lower risk of type 1 diabetes compared with other autoantibody pairs. In addition, we further assessed type 1 diabetes risk in all autoantibody pairs. It is evident that the prognostic implications for autoantibody combinations can be quite different between incident data from birth cohorts and prevalence data from screened cohorts.
The lower risk related to IAA/GADA positivity in screened cohorts compared with birth cohorts may not entirely be related to older age, since the median age of individuals with combined IAA and GADA in our cohort was less than 10 years. Intermolecular antigen spreading, specifically following IAA seroconversion, is thought to occur in early childhood [23, 29]. As the majority of individuals with IAA/GADA at mAb determination did not develop IA-2A or ZnT8A over the time of monitoring in our study, they may have already passed this antigen spreading time. Based on our results, among those with 2 autoantibodies, autoantibody positive pairs at baseline screening can predict risk of progression, providing a more personalised approach to clinical trial recruitment and facilitating recruitment of higher risk participants in prevention clinical trials.
Also, the similar risk for type 1 diabetes between those with 2 and those with >2 autoantibodies was notable. This differs from findings in other studies where individuals with increasing numbers of autoantibodies in the Diabetes Prevention Trial–Type 1 and in birth cohorts have the highest risk of progression [2, 5, 13]. The basis for this difference is not clear. A possible explanation is that once metabolic disturbances are present (e.g. elevated Index60 or dysglycaemia), the difference in risk between having 2 and >2 autoantibodies (from IAA, GADA, IA-2A and ZnT8A) is not significant. However, in the Diabetes Prevention Trial–Type 1 study, which showed a higher risk among those with >2 autoantibodies [19], individuals were screened for islet cell autoantibodies (ICA), which we excluded, and the autoantibody to ZnT8 had not been discovered at that time. Moreover, as mentioned above, birth cohorts can differ markedly from prevalence cohorts regarding the prognostic implications of autoantibodies.
Other characteristics of autoantibodies, besides their type, could influence the risk of type 1 diabetes. For example, it has become apparent that autoantibody affinity is a critical characteristic that should also be taken into account: single GADA and single IAA autoantibodies that are positive by electrochemiluminescence are indicative of much higher risk of type 1 diabetes than those that are negative [30,31,32]. Autoantibody titre also appears to affect the risk of type 1 diabetes [7, 18, 33, 34] and would be valuable data to add in future studies.
Measures of metabolic status, such as insulin, C-peptide, glucose, and combinations of C-peptide and glucose, have also been shown to be predictive of type 1 diabetes [11, 21, 22, 35]. For example, ICA-positive relatives of individuals with type 1 diabetes in the large European Nicotinamide Diabetes Intervention Trial (ENDIT) demonstrated clear augmentation of risk of progression to diabetes over 5 years based on dysglycaemia and the first phase insulin response [22]. In our analysis, we focused on Index60, a novel and composite marker of C-peptide and glucose, in addition to analysing a well-known metabolic marker (impaired blood glucose level). The presence of Index60 ≥1.0 or dysglycaemia placed participants at substantial risk for type 1 diabetes. Other risk factors, such as age, were less impactful in the presence of metabolic abnormalities.
The variability in risk of type 1 diabetes has been clearly demonstrated in this study in individuals with relatives who have type 1 diabetes, as well as in birth cohorts and smaller screened populations [5, 7, 14, 18, 20, 25, 27, 33]. In our study, we identified a subset of participants with low risk of progression based on age, autoantibody type and metabolic status. In comparison, using a combination of birth and screened cohorts across a broad age range but a smaller sample size, Long et al [36] did not demonstrate autoantibody differences in individuals characterised as slow progressors (diabetes-free 10 years after mAb determination). The Diabetes in Autoimmunity Study in the Young (DAISY) birth cohort identified mAb-positive slow progressors that were set apart from rapid progressors by IAA titre and the rate of progression from single to mAb positivity [7].
Assessing this risk of progression for potential enrolment in prevention trials is not without difficulty. However, the consideration of autoantibody types (beyond the number of autoantibodies) could itself provide a more refined and efficient selection of research participants, or at least improve the selection of those who should have metabolic testing. The change in autoantibodies over time has also been shown to impact risk, such as the loss of IAA [20].
Our study has certain limitations. The findings might not be fully representative of other cohorts screened for autoantibodies, especially if entry criteria differ from those of the TrialNet PTP study. Autoantibody titre and affinity, as well as genotype, were not assessed. Additionally, 5 year risk estimates are not necessarily indicative of the likelihood of long-term progression to type 1 diabetes.
This study also has certain strengths. The TrialNet PTP study has a large, diverse population that is sufficient in number for the more subtle subgroup analyses on which much of our findings were based. Also, participants in the TrialNet PTP have been well characterised with regard to both autoantibody and metabolic status.
It has been proposed that progression to type 1 diabetes should be viewed in terms of three stages, with the first being the development of mAbs, followed by dysglycaemia in the presence of mAbs and, finally, metabolic decompensation and onset of clinical diabetes [6]. Although this staging paradigm could be appropriate for many who have progressed to type 1 diabetes, our findings, in addition to previous works discussed here, suggest that this does not apply to all; i.e. individuals who have mAbs do not necessarily progress to type 1 diabetes. Importantly, our findings not only show that type 1 diabetes risk varies substantially in a population with mAbs, but that appreciable numbers of individuals who appear unlikely to progress to type 1 diabetes can be identified. With this knowledge, prevention clinical trials can better target those most likely to progress to type 1 diabetes and enrolment criteria can be designed with these features in mind.
Data availability
All data generated or analysed during this study are included in this published article or in the NIDDK Central Repository at https://repository.niddk.nih.gov/studies/trialnet/.
Abbreviations
- GADA:
-
Glutamic acid decarboxylase autoantibodies
- IAA:
-
Insulin autoantibodies
- IA-2A:
-
Insulinoma-associated antigen-2 autoantibodies
- ICA:
-
Islet cell autoantibodies
- mAb:
-
Multiple autoantibody
- PTP:
-
Pathway to Prevention
- TEDDY:
-
The Environmental Determinants of Diabetes in the Young
- ZnT8A:
-
Zinc transporter 8 autoantibodies
References
Ziegler AG, Hummel M, Schenker M, Bonifacio E (1999) Autoantibody appearance and risk for development of childhood diabetes in offspring of parents with type 1 diabetes: the 2-year analysis of the German BABYDIAB Study. Diabetes. 48(3):460–468. https://doi.org/10.2337/diabetes.48.3.460
Steck AK, Vehik K, Bonifacio E et al (2015) Predictors of progression from the appearance of islet autoantibodies to early childhood diabetes: The Environmental Determinants of Diabetes in the Young (TEDDY). Diabetes Care 38(5):808–813. https://doi.org/10.2337/dc14-2426
Vehik K, Lynch KF, Schatz DA et al (2016) Reversion of β-cell autoimmunity changes risk of type 1 diabetes: TEDDY Study. Diabetes Care 39(9):1535–1542. https://doi.org/10.2337/dc16-0181
ClinicalTrials.gov (2017) Type 1 Diabetes Prediction and Prevention (DIPP) Study (DIPP). Available from https://clinicaltrials.gov/ct2/show/NCT03269084. Accessed 2 February 2018
Ziegler AG, Rewers M, Simell O et al (2013) Seroconversion to multiple islet autoantibodies and risk of progression to diabetes in children. JAMA 309(23):2473–2479. https://doi.org/10.1001/jama.2013.6285
Insel RA, Dunne JL, Atkinson MA et al (2015) Staging presymptomatic type 1 diabetes: a scientific statement of JDRF, the Endocrine Society, and the American Diabetes Association. Diabetes Care 38(10):1964–1974. https://doi.org/10.2337/dc15-1419
Steck AK, Dong F, Waugh K et al (2016) Predictors of slow progression to diabetes in children with multiple islet autoantibodies. J Autoimmun 72:113–117. https://doi.org/10.1016/j.jaut.2016.05.010
Mahon JL, Sosenko JM, Rafkin-Mervis L et al (2009) The TrialNet Natural History Study of the Development of Type 1 Diabetes: objectives, design, and initial results. Pediatr Diabetes 10(2):97–104. https://doi.org/10.1111/j.1399-5448.2008.00464.x
Bonifacio E, Yu L, Williams AK et al (2010) Harmonization of glutamic acid decarboxylase and islet antigen-2 autoantibody assays for national institute of diabetes and digestive and kidney diseases consortia. J Clin Endocrinol Metab 95(7):3360–3367. https://doi.org/10.1210/jc.2010-0293
Yu L, Boulware DC, Beam CA et al (2012) Zinc transporter-8 autoantibodies improve prediction of type 1 diabetes in relatives positive for the standard biochemical autoantibodies. Diabetes Care 35(6):1213–1218. https://doi.org/10.2337/dc11-2081
Sosenko JM, Skyler JS, DiMeglio LA et al (2015) A new approach for diagnosing type 1 diabetes in autoantibody-positive individuals based on prediction and natural history. Diabetes Care 38(2):271–276. https://doi.org/10.2337/dc14-1813
Nathan BM, Boulware D, Geyer S et al (2017) Dysglycemia and Index60 as prediagnostic end points for type 1 diabetes prevention trials. Diabetes Care 40(11):1494–1499. https://doi.org/10.2337/dc17-0916
Vehik K, Haller MJ, Beam CA et al (2011) Islet autoantibody seroconversion in the DPT-1 Study - justification for repeat screening throughout childhood. Diabetes Care 34(2):358–362. https://doi.org/10.2337/dc10-1494
Jin Y, Sharma A, Bai A et al (2014) Risk of type 1 diabetes progression in islet autoantibody-positive children can be further stratified using expression patterns of multiple genes implicated in peripheral blood lymphocyte activation and function. Diabetes 63(7):2506–2515. https://doi.org/10.2337/db13-1716
Krischer JP, Lynch KF, Lernmark Å et al (2017) Genetic and environmental interactions modify the risk of diabetes-related autoimmunity by 6 years of age: The TEDDY Study. Diabetes Care 40(9):1194–1202. https://doi.org/10.2337/dc17-0238
Perry DJ, Wasserfall CH, Oram RA et al (2018) Application of a genetic risk score to racially diverse type 1 diabetes populations demonstrates the need for diversity in risk-modeling. Sci Rep 8:4529
Redondo MJ, Geyer S, Steck AK et al (2018) A type 1 diabetes genetic risk score predicts progression of islet autoimmunity and development of type 1 diabetes in individuals at risk. Diabetes Care 41(9):1887–1894. https://doi.org/10.2337/dc18-0087
Giannopoulou EZ, Winkler C, Chmiel R et al (2015) Islet autoantibody phenotypes and incidence in children at increased risk for type 1 diabetes. Diabetologia 58(10):2317–2323. https://doi.org/10.1007/s00125-015-3672-y
Orban T, Sosenko JM, Cuthbertson D et al (2009) Pancreatic islet autoantibodies as predictors of type 1 diabetes in the Diabetes Prevention Trial–Type 1. Diabetes Care 32(12):2269–2274. https://doi.org/10.2337/dc09-0934
Gorus FK, Balti EV, Messaaoui A et al (2017) Twenty-year progression rate to clinical onset according to autoantibody profile, age, and HLA-DQ genotype in a registry-based group of children and adults with a first-degree relative with type 1 diabetes. Diabetes Care 40(8):1065–1072. https://doi.org/10.2337/dc16-2228
Xu P, Beam CA, Cuthbertson D et al (2012) Prognostic accuracy of immunologic and metabolic markers for type 1 diabetes in a high-risk population: receiver operating characteristic analysis. Diabetes Care 35(10):1975–1980. https://doi.org/10.2337/dc12-0183
Bingley PJ, Gale EAM, The European Nicotinamide Diabetes Intervention Trial (ENDIT) Group (2006) Progression to type 1 diabetes in islet cell antibody-positive relatives in the European Nicotinamide Diabetes Intervention Trial: the role of additional immune, genetic and metabolic markers of risk. Diabetologia 49(5):881–890. https://doi.org/10.1007/s00125-006-0160-4
Bosi E, Boulware DC, Becker DJ et al (2017) Impact of age and antibody type on progression from single to multiple autoantibodies in type 1 diabetes relatives. J Clin Endocrinol Metab 102(8):2881–2886. https://doi.org/10.1210/jc.2017-00569
Gorus FK, Balti EV, Vermeulen I et al (2013) Screening for insulinoma antigen 2 and zinc transporter 8 autoantibodies: a cost-effective and age-independent strategy to identify rapid progressors to clinical onset among relatives of type 1 diabetic patients. Clin Exp Immunol 171(1):82–90
Endesfelder D, Zu Castell W, Bonifacio E et al (2019) Time-resolved autoantibody profiling facilitates stratification of preclinical type 1 diabetes in children. Diabetes 68(1):119–130. https://doi.org/10.2337/db18-0594
Bingley PJ, Boulware DC, Krischer JP (2016) Type 1 Diabetes TrialNet Study Group. The implications of autoantibodies to a single islet antigen in relatives with normal glucose tolerance: development of other autoantibodies and progression to type 1 diabetes. Diabetologia 59(3):542–549. https://doi.org/10.1007/s00125-015-3830-2
Decochez K, De Leeuw IH, Keymeulen B et al (2002) IA-2 autoantibodies predict impending type I diabetes in siblings of patients. Diabetologia 45(12):1658–1666. https://doi.org/10.1007/s00125-002-0949-8
Krischer JP, Liu X, Vehik K et al (2019) Predicting islet cell autoimmunity and type 1 diabetes: an 8-year TEDDY Study progress report. Diabetes Care 42(6):1051–1060
Brooks-Worrell B, Gersuk VH, Greenbaum C, Palmer JP (2001) Intermolecular antigen spreading occurs during the preclinical period of human type 1 diabetes. J Immunol 166(8):5265–5270
Fouts A, Pyle L, Yu L et al (2016) Do electrochemiluminescence assays improve prediction of time to type 1 diabetes in autoantibody-positive TrialNet subjects? Diabetes Care 39(10):1738–1744. https://doi.org/10.2337/dc16-0302
Steck AK, Fouts A, Miao D et al (2016) ECL-IAA and ECL-GADA can identify high-risk single autoantibody-positive relatives in the TrialNet Pathway to Prevention Study. Diabetes Technol Ther 18(7):410–414. https://doi.org/10.1089/dia.2015.0316
Sosenko JM, Yu L, Skyler JS et al (2017) The use of electrochemiluminescence assays to predict autoantibody and glycemic progression toward type 1 diabetes in individuals with single autoantibodies. Diabetes Technol Ther 19(3):183–187. https://doi.org/10.1089/dia.2016.0243
Achenbach P, Warncke K, Reiter J et al (2004) Stratification of type 1 diabetes risk on the basis of islet autoantibody characteristics. Diabetes 53(2):384–392. https://doi.org/10.2337/diabetes.53.2.384
Sosenko JM, Skyler JS, Palmer JP et al (2013) The prediction of type 1 diabetes by multiple autoantibody levels and their incorporation into an autoantibody risk score in relatives of type 1 diabetic patients. Diabetes Care 36(9):2615–2620. https://doi.org/10.2337/dc13-0425
Xu P, Wu Y, Zhu Y et al (2010) Prognostic performance of metabolic indexes in predicting onset of type 1 diabetes. Diabetes Care 33(12):2508–2513. https://doi.org/10.2337/dc10-0802
Long AE, Wilson IV, Becker DJ et al (2018) Characteristics of slow progression to diabetes in multiple islet autoantibody-positive individuals from five longitudinal cohorts: the SNAIL study. Diabetologia 61(6):1484–1490. https://doi.org/10.1007/s00125-018-4591-5
Acknowledgements
We acknowledge the support of the Type 1 Diabetes TrialNet Study Group, which identified study participants and provided samples and follow-up data for this study. The authors also acknowledge M. Warnock (TrialNet statistician; Tampa, FL, USA) and the TrialNet Coordinating Center for their assistance and support. Parts of this study were presented in oral presentation at the 77th Scientific Sessions of the American Diabetes Association, San Diego, CA, USA (9–13 June 2017).
Funding
The Type 1 Diabetes TrialNet Study Group is a clinical trials network funded by the National Institutes of Health (NIH) through the National Institute of Diabetes and Digestive and Kidney Diseases, the National Institute of Allergy and Infectious Diseases, and the Eunice Kennedy Shriver National Institute of Child Health and Human Development, through cooperative agreements U01 DK061010, U01 DK061034, U01 DK061042, U01 DK061058, U01 DK085465, U01 DK085453, U01 DK085461, U01 DK085466, U01 DK085499, U01 DK085504, U01 DK085509, U01 DK103180, U01 DK103153, U01 DK085476, U01 DK103266, U01 DK103282, U01 DK106984, U01 DK106994, U01 DK107013, U01 DK107014, UC4 DK106993, and the JDRF. The contents of this article are solely the responsibility of the authors and do not necessarily represent the official views of the NIH or the JDRF.
Author information
Authors and Affiliations
Contributions
LMJ analysed the data and wrote the manuscript. LB, DB and SG analysed the data, contributed to discussion and provided statistical guidance. CEM, LD, RG, DMW, MAA, TA, WER, JMW, SG and DB were involved in the acquisition and interpretation of data, as well as revising the manuscript critically for important intellectual content. LB and JMS assisted in drafting and revising the manuscript. All authors have provided final approval of the version to be published. JMS conceptualised this study and is the guarantor of this work and, as such, had full access to all the data in the study and takes responsibility for the integrity of the data and the accuracy of the data analysis.
Corresponding author
Ethics declarations
The authors declare that there is no duality of interest associated with this manuscript.
Additional information
Publisher’s note
Springer Nature remains neutral with regard to jurisdictional claims in published maps and institutional affiliations.
Electronic supplementary material
ESM
(PDF 504 kb)
Rights and permissions
About this article
Cite this article
Jacobsen, L.M., Bocchino, L., Evans-Molina, C. et al. The risk of progression to type 1 diabetes is highly variable in individuals with multiple autoantibodies following screening. Diabetologia 63, 588–596 (2020). https://doi.org/10.1007/s00125-019-05047-w
Received:
Accepted:
Published:
Issue Date:
DOI: https://doi.org/10.1007/s00125-019-05047-w